Yesterday I completed my 'Learn what the Data School learns' lesson on the Fixed LOD calculation in Tableau. As an introductory lesson to LOD's the basis of the lesson revolved around the concept of granularity and how LOD calculations are related, which I will be going over in this blog.
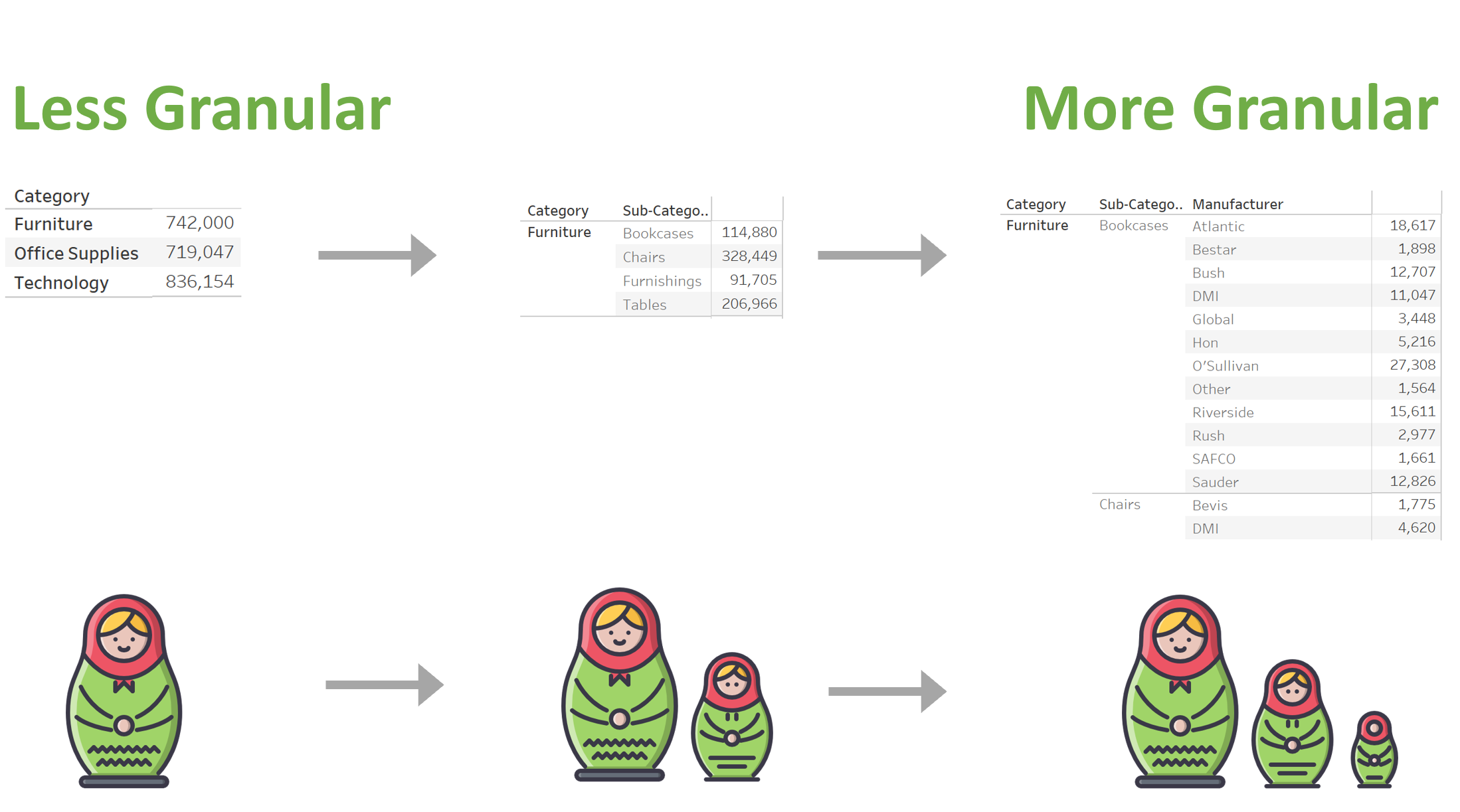
I've always thought of granularity as 'distance' from the row level of a given data set. The more granular the closer we are to specifying a specific row. This change in granularity also goes through it's correlated sub-sector, in the example above it's shown via Category -> Sub-Category -> Manufacturer. Each is contained within the previous, hopefully the Russian doll analogy helps to show this.
What is a Fixed LOD?
A { FIXED } LOD calculation is performed at an independent level by FIXING the level of detail in the calculation, regardless of the current granularity in the view. Essentially, the Fixed LOD allows you to reference a different 'level' of granularity than what's currently in the view, unlike Table Calcs which can't perform this task. A very useful tool for comparisons.
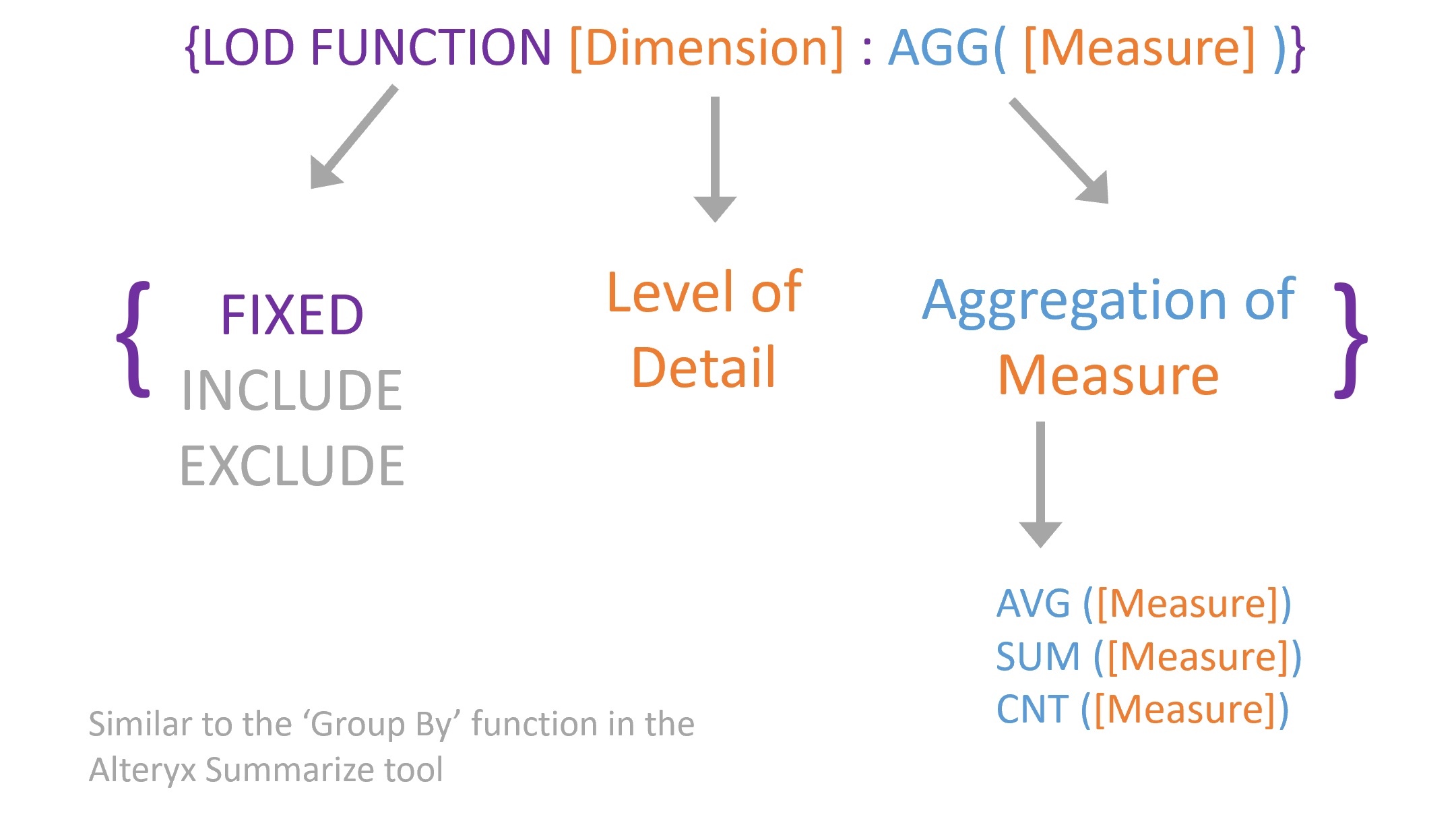
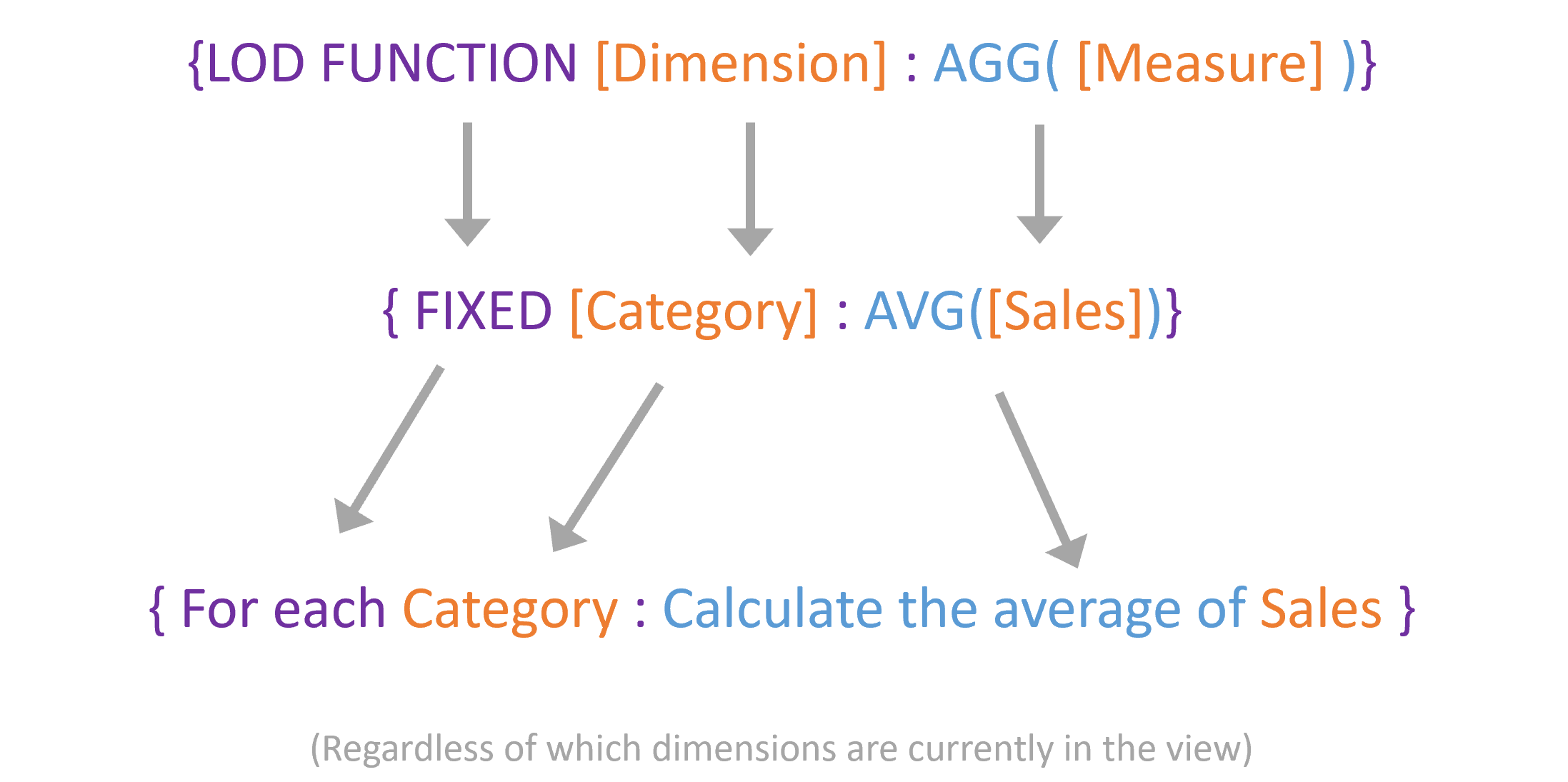
Using the syntax above, we can compare a calculation in relation to category (less granular, not in the view) to manufacturers (more granular, currently in the view) as shown below:
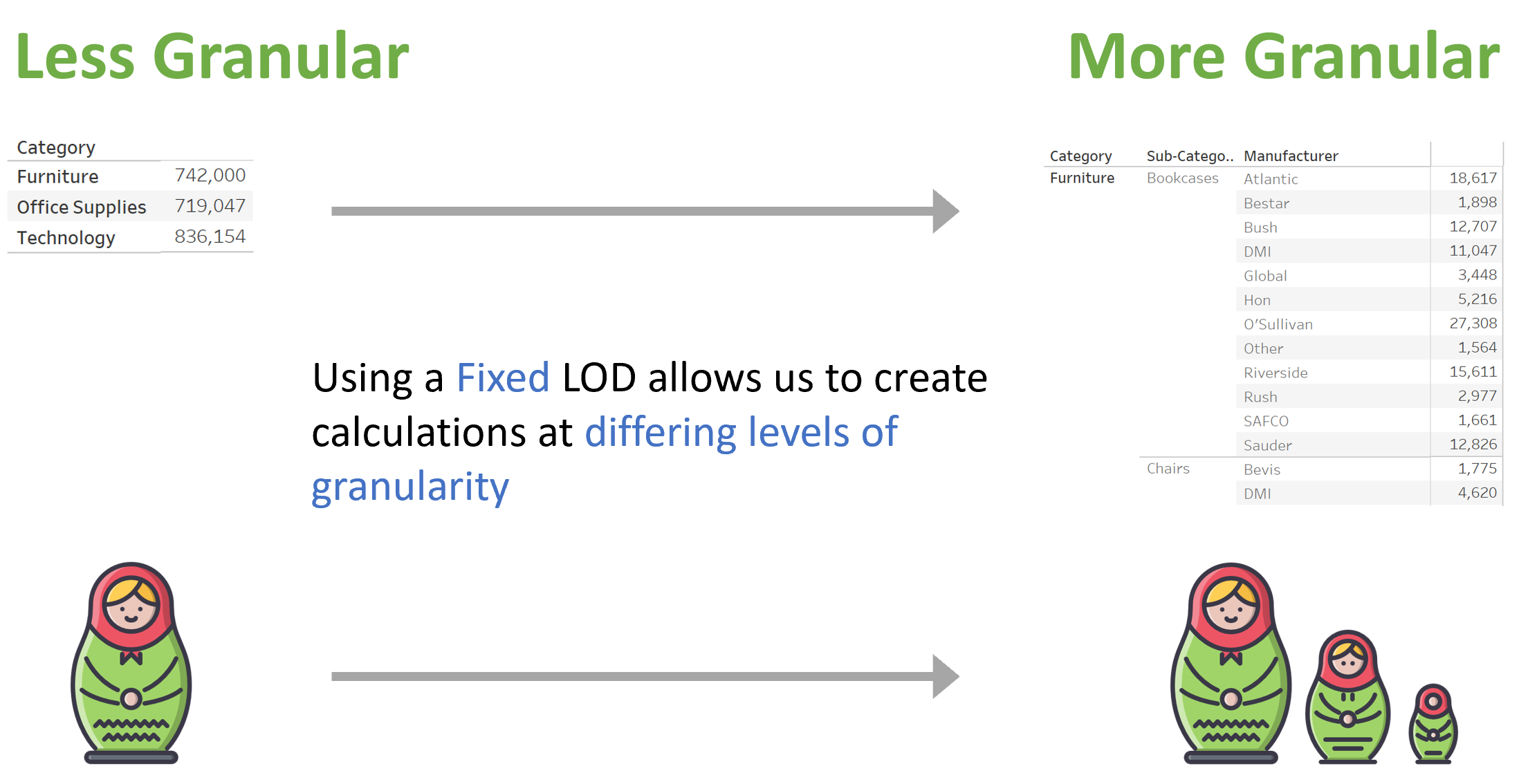
Finally, the example below shows the FIXED LOD in Tableau using Category and Sales:
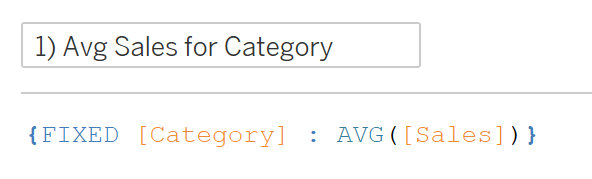
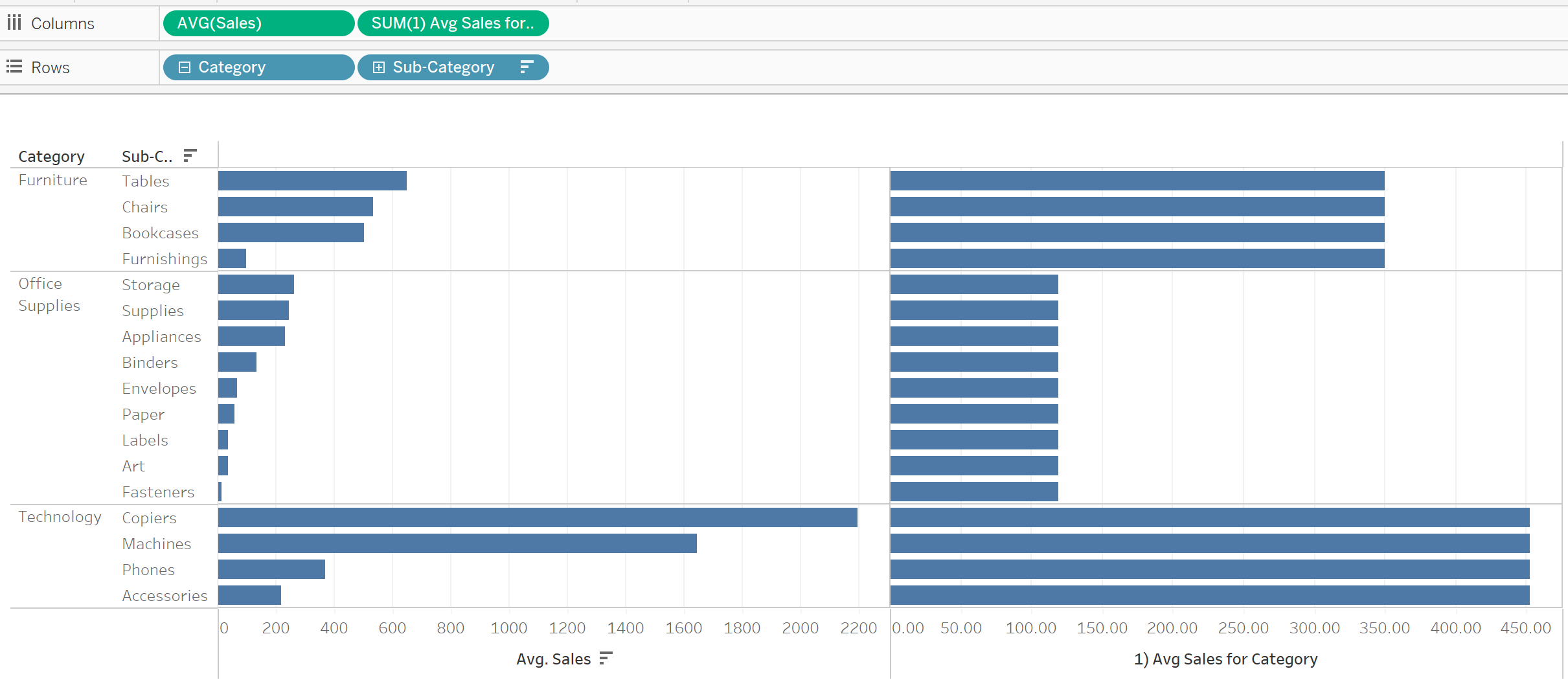
Using our Fixed LOD we can compare our average sales for a given sub-category to average sales within the whole category it resides in:
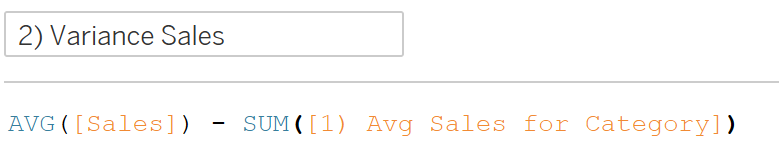
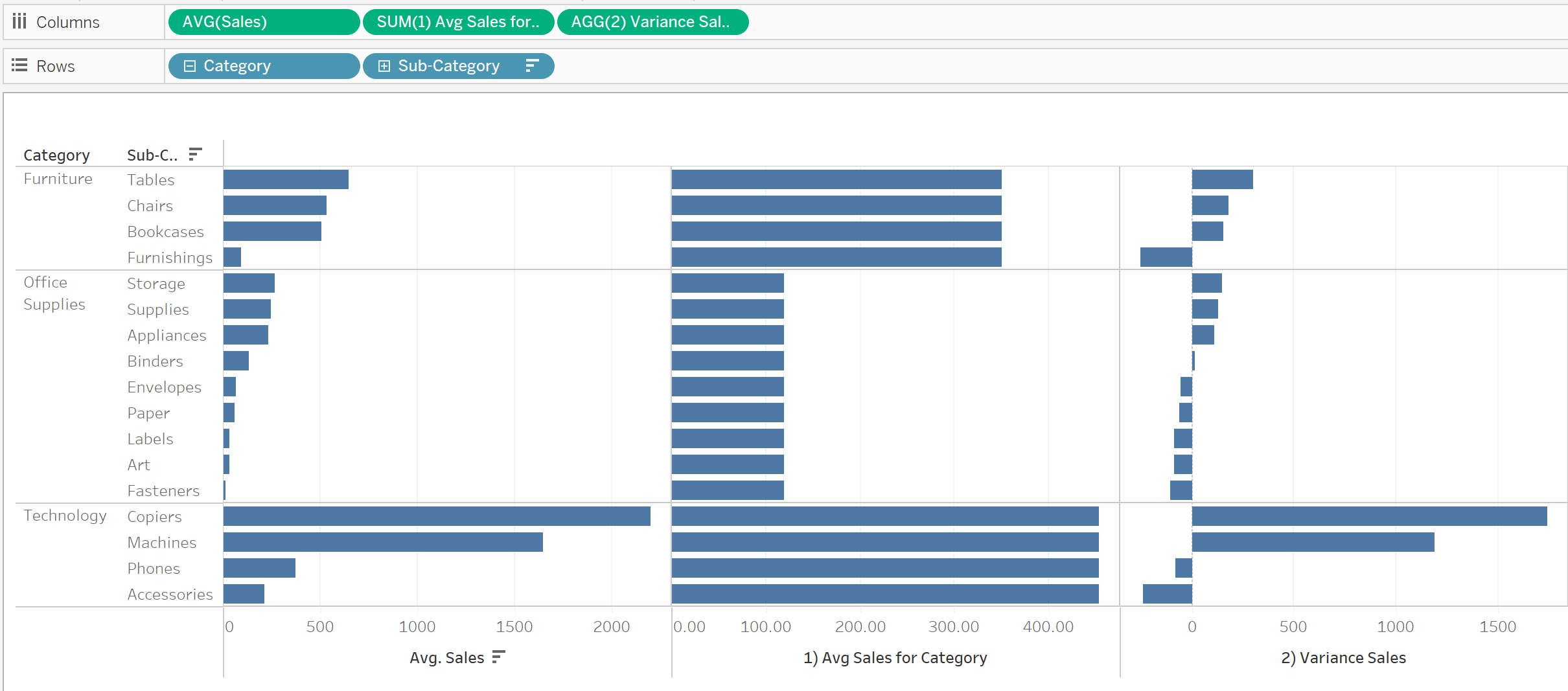
This is just the tip of the iceberg for LOD calculations, but understanding it's relationship to granularity is the first step to understanding them. My full lesson can be found on TIL YouTube channel, or tune into DS30's Learn what the Data School learns in a couple of weeks for a live session on similar topics!