Welcome to my first blog post as a Data School Consultant. The aim of these blog posts is to help us reflect on what we have learned and experienced over the weeks. In our first week at the begginning of august, we have already learned many exciting things, such as how to use Tabelau Prep or how to prepare our work so that it is as comprehensible and usable as possible for others. However, I think the lesson that stuck with me, especially in the first week, is that it's super important to know the basics.
What do I mean by that? Quite simply, we always talk a lot about data. But of course it is important to know what kind of data we have in front of us and in what form it is available in order to decide how the data can be processed efficiently and which tool to use for this. And of course I don't know how you feel about the terms database, data warehouse, data lake and data lake house, but I was initially quite confused. I would therefore like to visualize an example with you here that has made the distinction clearer to me. Let's start with the database
Database:
A database is a collection of data that is typically structured and has only one source. In contrast to the other data variants, they are optimized for data accessibility and retrieval. The database is usually only relevant for one application or one organization.
Example:
As an analogy, you can imagine a database as a bookshelf that is structured by alphabet. A book in this example represents different database tables.
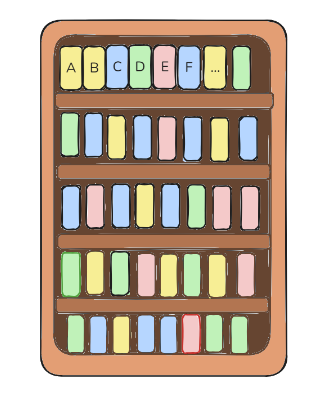
Data Warehouse:
A data warehouse is a unified data repository for storing large amounts of information from multiple sources within an organization. Structured Data Warehouses extract data from multiple sources and transform and clean the data before loading it into the warehouse system to serve as a single source of data warehouses. Store clean and relational data.
Example:
A library in which books from various sources, such as book donations, book purchases, etc., end up and are then structured in the many different book shelves, e.g. sorted by genre, can be described as a data warehouse.
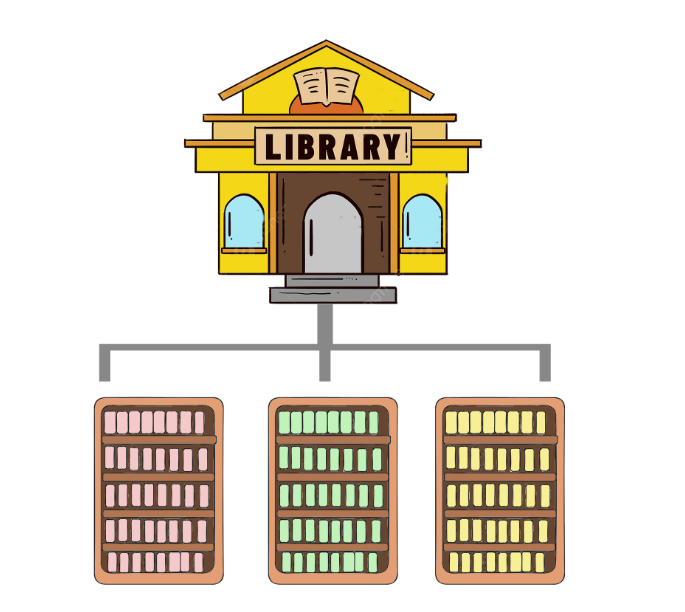
Data Lakes
Data Lake is a centralized, highly flexible storage repository that stores large amounts of unstructures and unstructures data in its raw, original and unformatted form. They allow for machine learning and predictive analysis.
Example:
In contrast, a library in which books are not sorted but simply lie around in an unstructured heap (book lake) could be described as a data lake.
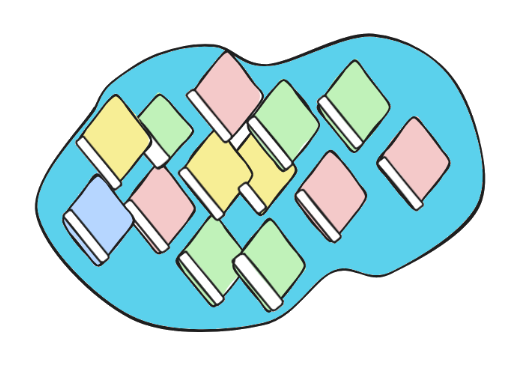
Data lakehouse
A data lakehouse is a combination of the data warehouse and the data lake that combines their advantages (organized data sets with structured data and large repositories of raw data in its original form). This means, for example, that structured data for e.g. business intelligence no longer has to be stored in a separate location from the semi-structured and unstructured data in a data lake. This reduces complexity, higher costs and avoids problems in terms of data consistency, duplication and costs.
Example:
So imagine a paradise for bookworms were the structured library is located at the same spot as the adjoining book lake. A bookworm doesn’t have to switch location to access the lake or vice versa.
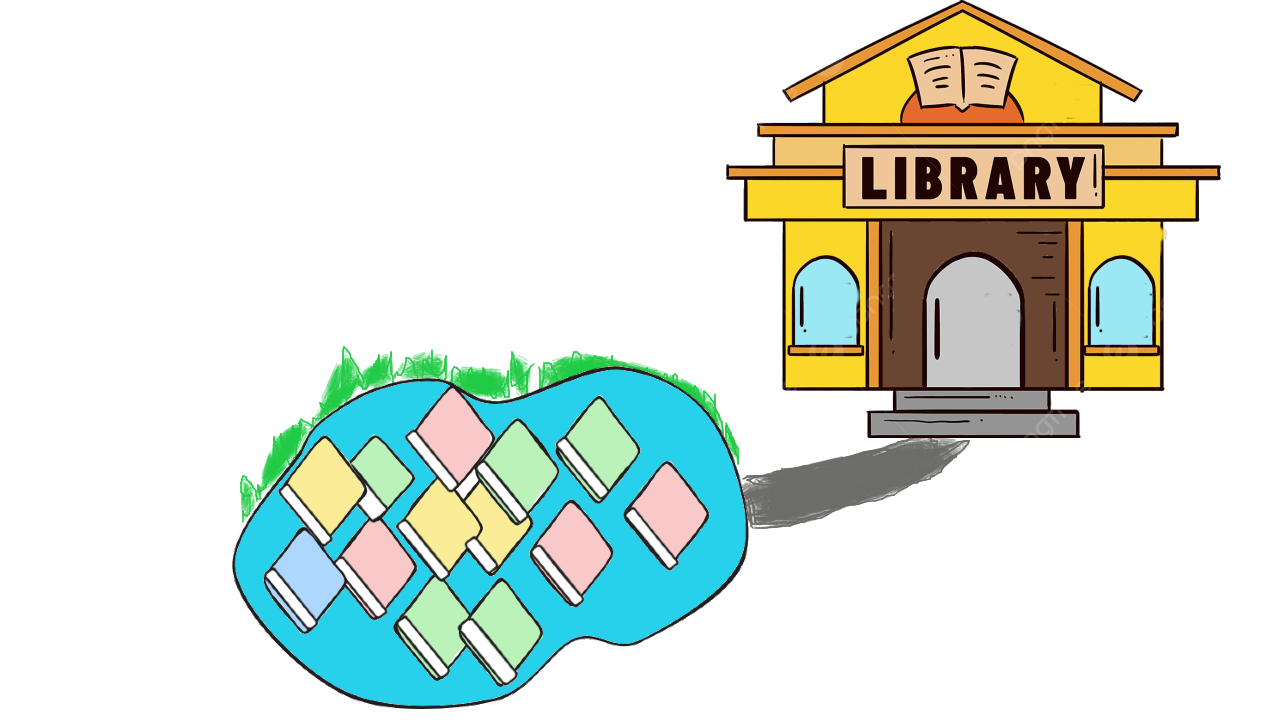