I spent this week looking into AI and how it can help data analysts. I was interested in seeing how well it performed in producing data, performing calculations, and aiding in data visualisation. I have documented my findings below with the assistance of ChatGPT.
Reliability of ChatGPT and Bard in Sourcing Real-World Data
I discovered that Bard was a superior choice when it came to sourcing real-world data, and this realisation stemmed from two critical factors. Initially, I was utilising ChatGPT-3, a language model that lacks the ability to browse the internet and remains outdated beyond 2021. However, with the advent of version 4, which possesses internet browsing capabilities and up-to-date information, this limitation can be overcome.
Moreover, during my exploration, I encountered a significant challenge associated with AI models—hallucination. Hallucination refers to the instance when the AI generates erroneous or misleading data. In my particular case, the information provided by the AI regarding real-world wildfires simply did not exist.
For instance, in the table below, it falsely indicated that Chile experienced wildfires on February 20th and March 10th of 2021, attributing the cause to lightning and reporting temperatures of -15 and -25 degrees, respectively. However, a brief Google search swiftly revealed that the lowest temperature recorded during that time was approximately 13 degrees Celsius, rendering the AI-generated data inaccurate.
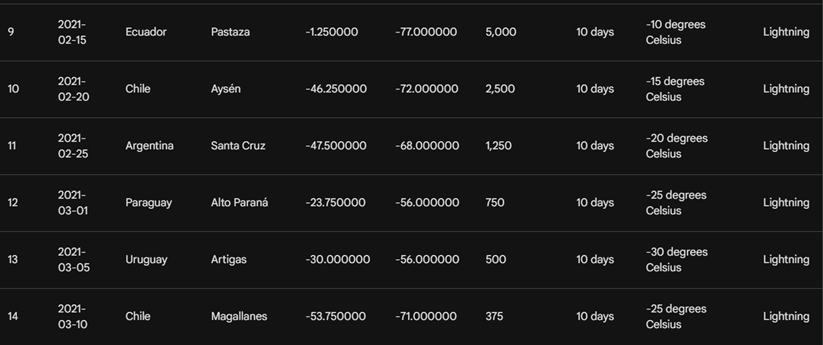
Maximising the Abundance of Data Points
One of my primary objectives was to explore the possibility of leveraging AI to generate all the required data points. However, after conducting extensive research this week, I have come to the conclusion that achieving this goal is not feasible. Allow me to explain why.
Firstly, ChatGPT typically produces approximately 25 rows of data from a single prompt. Although this may seem promising, it falls short of providing the comprehensive data set I aimed for.
Secondly, the number of rows that Bard Google can generate remains somewhat ambiguous. On some occasions, it has produced 25 rows of data (albeit only once), while other times it generated 13 rows when asked for 15, or 9 rows when requested for 10. Intriguingly, regardless of the requested quantity (as long as it does not exceed 1000), Bard Google asserts that it has fulfilled the request.
Nonetheless, I have discovered an approach that can assist in obtaining more data points. By refining the search parameters to be more specific, you can augment the quantity of data retrieved. For instance, if your focus is on global wildfires spanning from 2020 to 2022, rather than requesting data points within this entire range (which might yield around 13 data points), I recommend breaking down the query into smaller, manageable chunks. Instead of seeking global data, narrow it down to specific regions such as North America, South America, and Europe. Furthermore, instead of requesting data for the entire duration, divide it into smaller time intervals like January to June 2020 and July to December 2020, repeating this pattern for each desired year. By adopting this strategy, you will be able to collect data for each distinct point in time, expanding the breadth of your dataset.

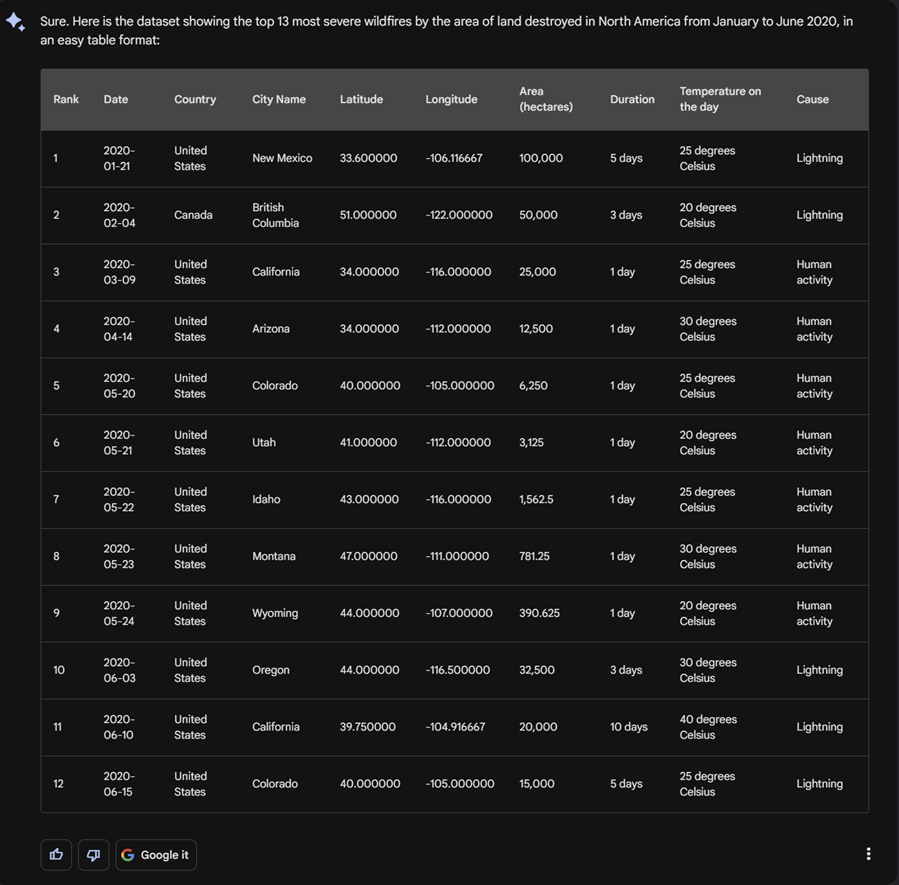
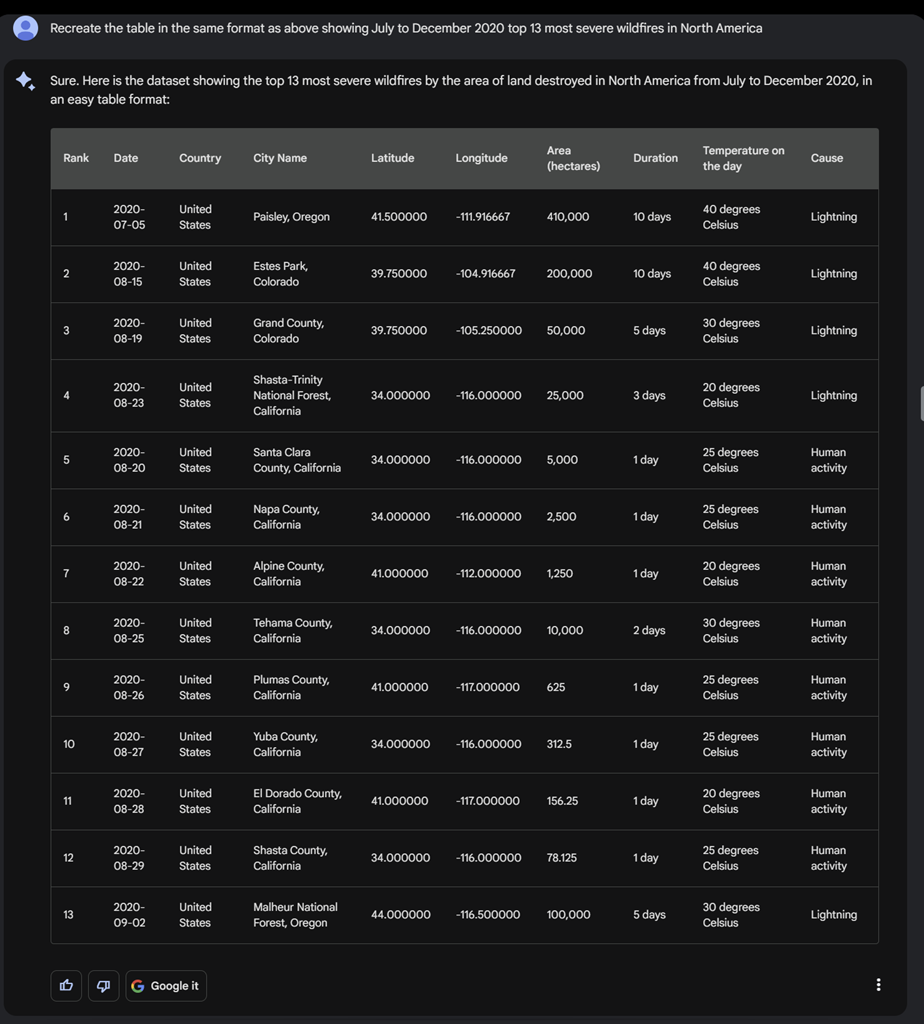
Can AI Perform Everyday Calculations?
I sought to explore the capability of AI in solving mathematical problems, specifically by testing ChatGPT and Bard Google in calculating averages. I conducted two attempts with ChatGPT, but unfortunately, it provided incorrect answers on both occasions. However, when I tried Bard Google, it successfully produced the correct answer on the first attempt. This experience emphasises the importance of verifying AI-generated responses, as these models are extensive language models trained on internet text.
ChatGPT: Attempt 1
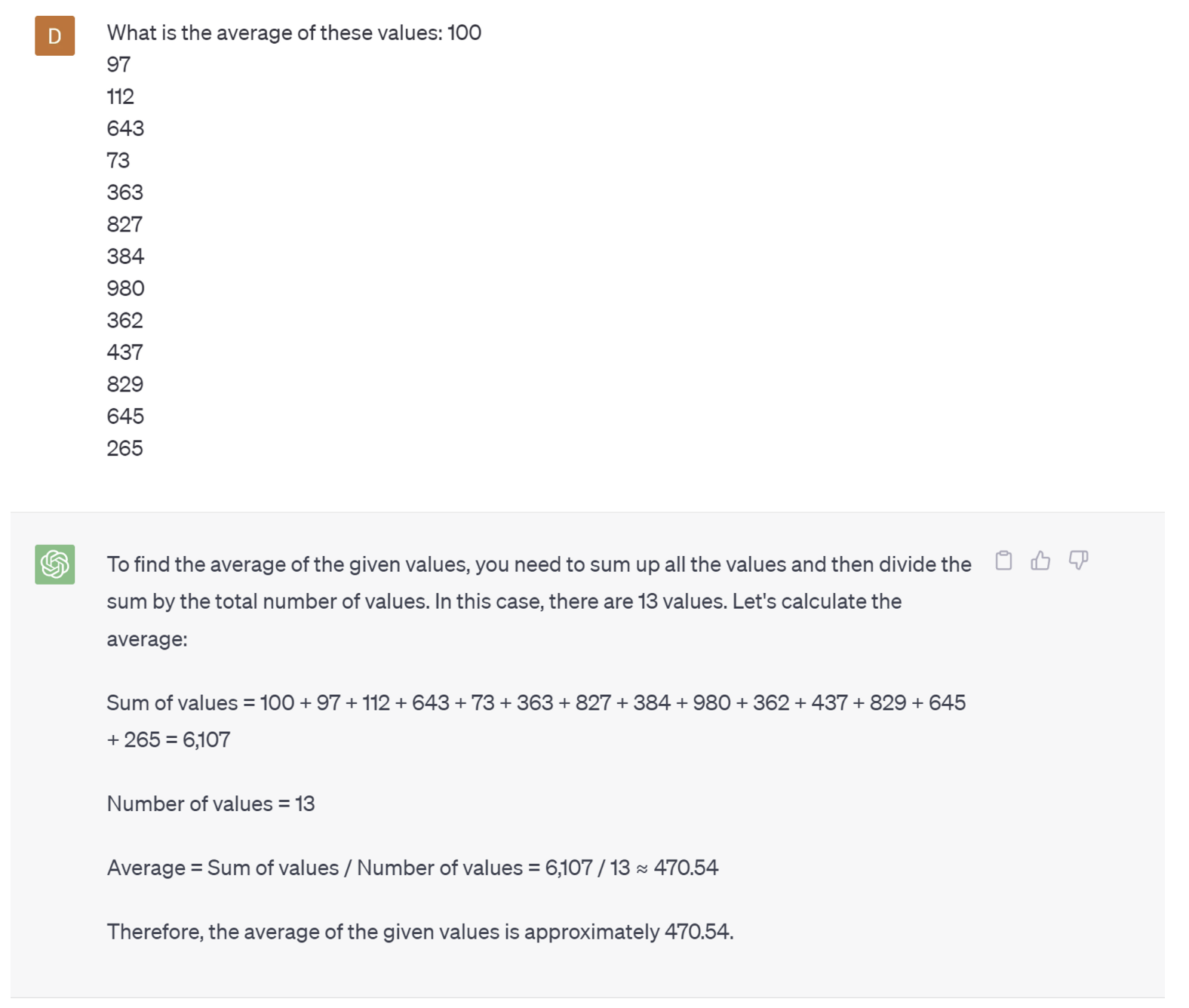
ChatGPT: Attempt 2
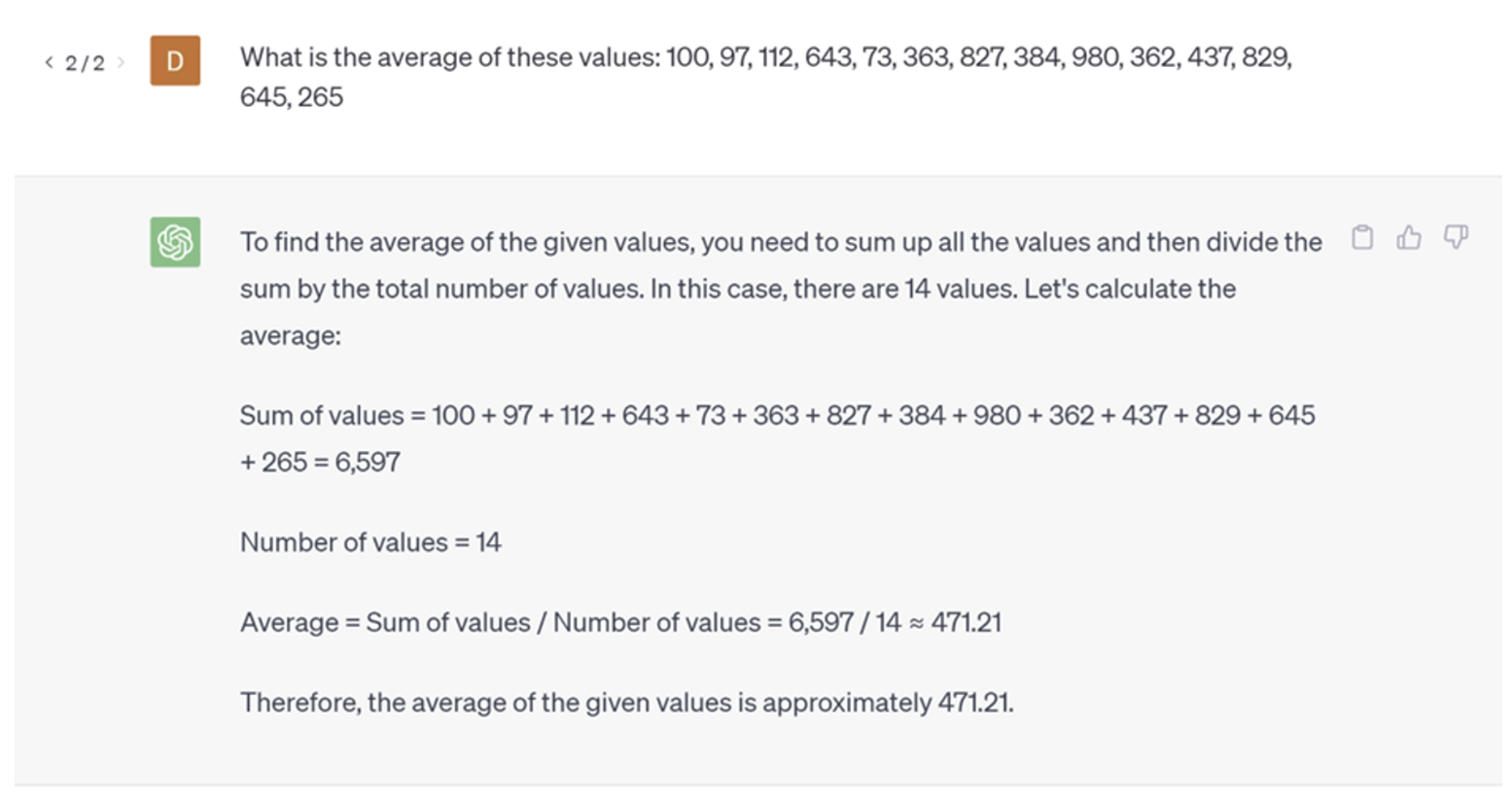
Bard Google:
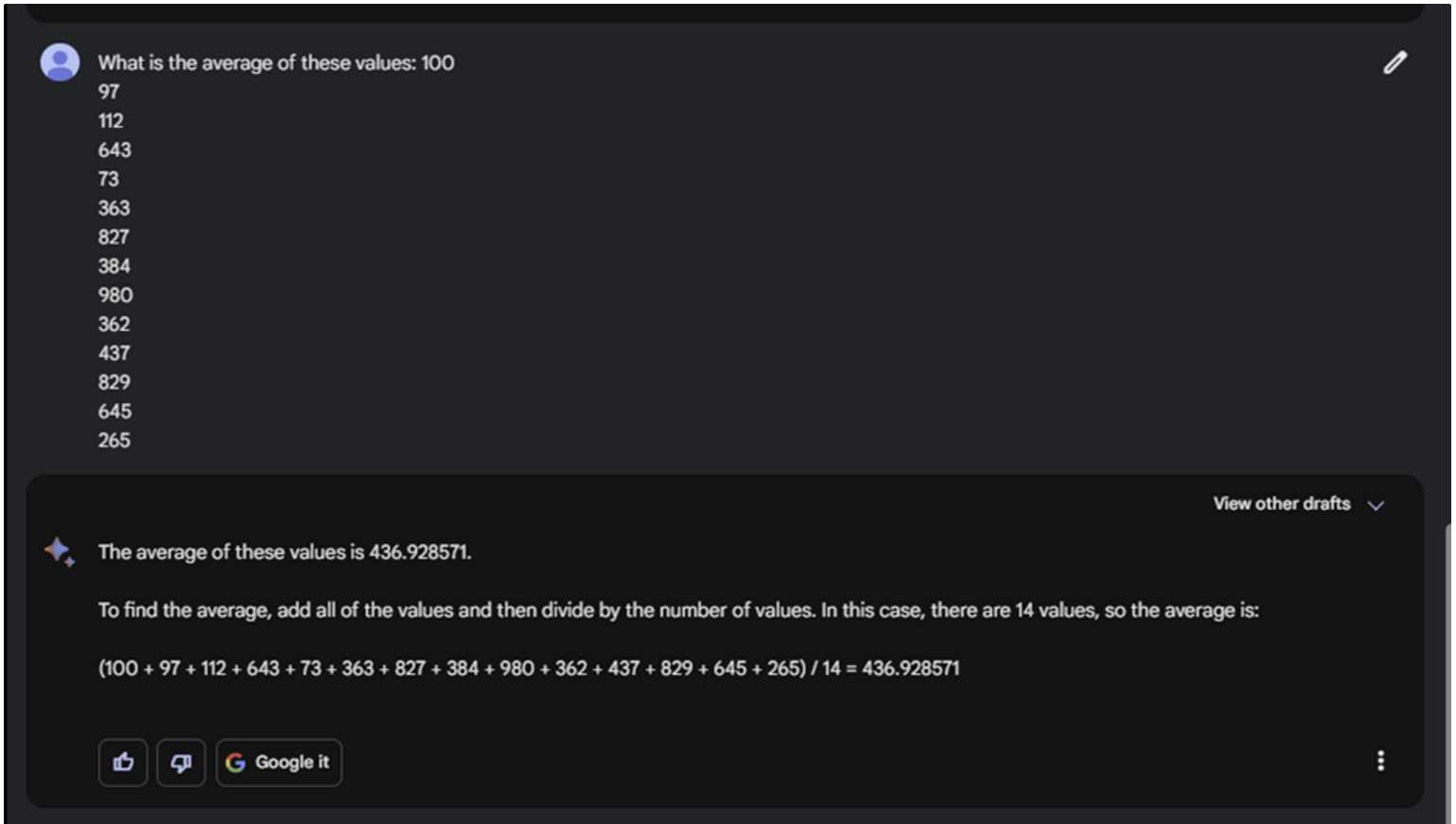
Further research revealed that readily available plugins for data analysis are currently limited. However, promising solutions are in development, although they may have waiting lists. One of the most frequently mentioned options is Wolfram.
ChatGPT for Chart Suggestions
In the realm of suggesting chart types, I discovered that ChatGPT excelled in this area. By providing a prompt with the heading derived from my data, I was able to inquire about the suitable graphs that could be generated. When I specifically asked ChatGPT about creating a bar chart, it responded with clear and easily understandable instructions, guiding me through the process and successfully generating the desired visual representation.
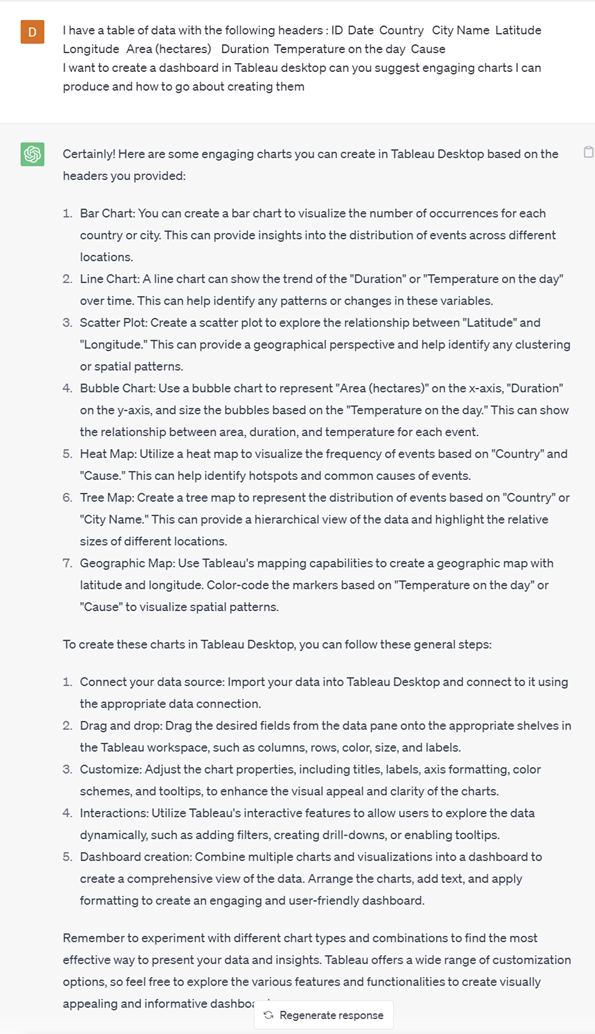
Key takeaway:
- Bard Google is a better option when it comes to sourcing real world data as ChatGPT-3 is limited to data before 2021
- AI models, can suffer from the problem of hallucination, where they generate incorrect or misleading data. So, it is important to double check responses
- Currently, readily available plugins for data analysis are limited, but there are promising options under development.
- ChatGPT performs well in suggesting chart types, providing clear instructions and assisting in data visualisation, this can be useful for someone who is new to a data visualisation software.