This blog is about the book "The Signal and the Noise" by Nate Silver published in the year 2012.
This week we started with Alteryx. After almost two and a half days of intensive training, we attempted our first Alteryx Core certification. Alteryx requires one month of practical experience. What was the likelihood of successfully completing the exam?
There are few things better suited to the pain the day after than reading the book "The Signal and the Noise". The title should definitely not put you off. This book is not about technology, but very clearly about right and wrong predictions and how we can improve our predictions. How can we separate the signals that interest us from the interference/noise? Why can some people make excellent predictions while the majority of supposed experts are completely wrong? Nate Silver gives countless wonderful examples to illustrate when and why predictions went wrong. He goes into great depth and throughout the book provides an understanding of we can fail when measuring and predicting, why this happens and how we can improve our predictions. Among other things, it deals with:
- the everlasting problem of the lack of causality of correlations;
- the problem of obtaining accurate measurement data, e.g. for weather forecasts or economic forecasts (dynamic systems);
- the danger of extrapolation, e.g. with exponentially growing populations and
- the problem of overfitting when we consider noise to be a signal and much, much more.
Of course, I cannot reproduce the numerous examples in the book here. The spectrum is enormous and ranges from driving, baseball, basketball, earthquakes and poker to chess computers, swine flu, terrorist attacks, weather forecasts, economic collapses and much more. I can only give you a few impressions here, which I hope will make you want to read the book for yourself. Believe me, it's worth it.
Some examples (from page 216 onwards) show that predictions can destroy or fulfill themselves. Do you always follow the fastest route with your gps? What happens if everyone does? Of course, then it might get very crowded while you're still on the route and the prediction will have destroyed itself. If the fashion industry predicts that the color brown will be the hottest trend next year, then many fashionistas will probably buy brown clothes and the prediction will have fulfilled itself.
In another example, Nate Silver reports (from page 223) that there were two outbreaks of measles in Chicago in the 1980s and 1990s. Although measles was considered one of the best-studied and best-known diseases, the two outbreaks were not covered by the predictions. Traditional models had instead predicted that enough Chicagoans would be vaccinated to achieve herd immunity. A term we are more than familiar with since Covid 19. Nevertheless, thousands of people, mostly children, contracted measles. The problem was that the usual spread models for measles assumed a uniform random distribution of encounters throughout the population. However, there were population groups that were both less vaccinated than the population as a whole and remained among themselves. Within these groups, the disease spread much faster than was predicted for the population as a whole. The same phenomenon also occurred under different conditions in other epidemics or disease outbreaks. In each case, the underlying model was oversimplified.
Silver makes it clear how useless data is without context (page 254) and points out countless weaknesses in our models and forecasts. Nate Silver sees a possible basis for improvements in Bayes' theory. Nate Silver advises us to think like Bayes in order to avoid false positives, for example. Silver illustrates the "Bayes theorem" very clearly, which deals with conditional probability, i.e. the probability that our hypothesis is true under the condition that an event has occurred. For example, how likely is it that your partner has cheated if you find an unknown piece of underwear under your own clothes after a business trip? How likely is it if this happens twice in a row? I'm not going to solve this here because I don't know your boundary conditions. You can find examples of calculations from page 248 onwards in the book.
This book has once again made one thing very clear to me. Good forecasts are not the result of ever-improving software tools or faster computers. They are created in our heads and require a lot of care.
How would Bayes have predicted my result in the Alteryx Core Exam?
Interesting Sources
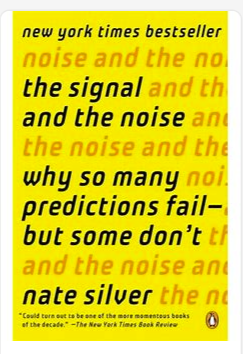